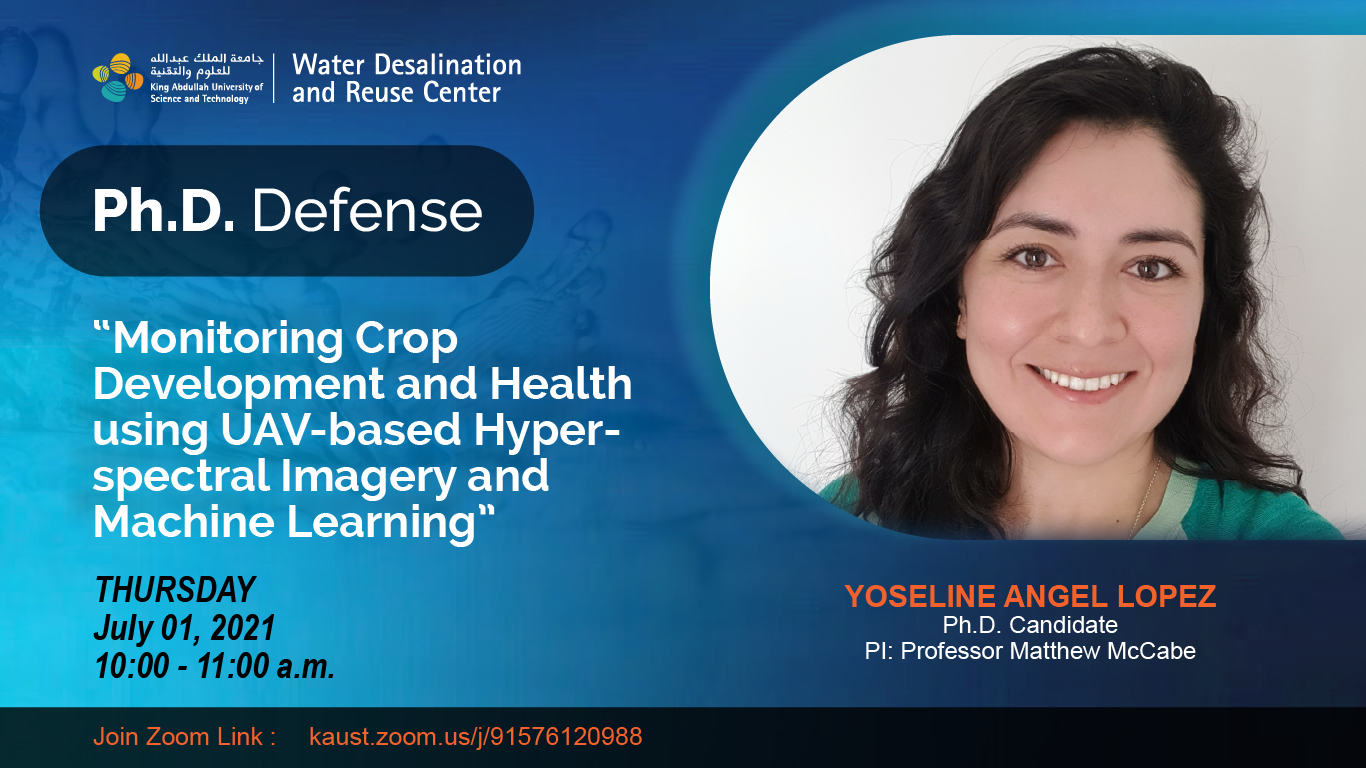
Monitoring Crop Development and Health using UAV-based Hyperspectral Imagery and Machine Learning
Abstract:
Agriculture faces many challenges related to the increasing food demands of a growing global population and the sustainable use of resources in a changing environment. To address such challenges, we need reliable information sources, particularly those that can help improve the monitoring and assessment of our agricultural systems. A potential source of such information lies in exploiting passive satellite, airborne, and ground-based remote sensing data to observe phenological traits through the crop's growth cycle and gather information to precisely diagnose when, why, and where a crop is suffering disease, abiotic stress, or other negative impacts. Large-scale hyperspectral imaging has been used for many years as a non-destructive mechanism to characterize crop physiology and describe plant-environment interactions. Indeed, spectrometers have long been used to measure plant reflectance across hundreds of visible (VIS) and near-infrared (NIR) wavelengths, which can be correlated with biophysical and biochemical characteristics, enhancing crop health monitoring and condition.
Here, a UAV-based hyperspectral solution for mapping crop physiological parameters was explored within a machine learning framework. To do this, a range of complementary measurements were collected from a field-based phenotyping experiment, based on a diversity panel of wild tomato (Solanum pimpinellifolium) that were grown under both fresh and saline conditions. The outcomes of this research illustrate the potential of UAV-based hyperspectral imaging for high-throughput plant phenotyping, precision agriculture, and environmental monitoring. In particular, this research a) developed systematic calibration and pre-processing workflows for producing spatially and spectrally accurate data cubes; b) explored a range of machine learning-driven tools for retrieving plant phenological dynamics; c) established a plant stress detection approach from hyperspectral-derived metrics; and d) provided new insights into using computer vision algorithms, big-data analytics, and modeling strategies to deal effectively with the complexity of the UAV-based hyperspectral data in mapping indicators that characterize specific physiological traits.
Short bio:
Yoseline studied Cadastral Engineering and Geodesy at the District University ‘Francisco Jose de Caldas’ in Bogota, Colombia. She spent 2 years as an associate academic researcher for the project "Integrated Illicit Crops Monitoring System (SIMCI)" at the United Nations Office on Drugs and Crime. In 2012, Yoseline received her MSc degree in Geomatics from the National University of Colombia (UNAL). She was a lecturer of remote sensing and photogrammetry courses at the District University in 2012. For 9 years, between 2006 and 2015, Yoseline worked as a Geographical Information System Specialist for Occidental Petroleum Corporation (OXY). In early 2016, she joined KAUST as Ph.D. Student in the Hydrology and Land Observation (HALO) group to advance her research in "Monitoring crop development and health using UAV-based hyperspectral imagery and machine learning," completing her Ph.D. in summer 2021. From October 2021, Yoseline will continue her academic career as a postdoctorate in the NASA Goddard Space Flight Center (GSFC).